I decided to start a new series that focuses on things that, in my opinion, does not get recognized during or after introduction to statistics class. The main focus of the series will be on stuff that are not mentioned when a person gets stats class for the first time. This and upcoming pieces will be based on books like OpenIntro Stats by Mine Çetinkaya-Rundel, Learning Statistics by Daniel Navarro, Improving Your Statistical Inferences by Daniel Lakens. Let's go.
In a classic intro to stats, the class consist of two parts: Probability part and statistics part. But usually the difference between the two goes unmentioned.
I believe there to be a somewhat of a folk probability — everybody has some inherent understanding of what probability is. Probability is branch of mathematics that is dealing with chances. Questions like "how likely I am to observe 8 heads in 10 fair coin toss" or "what is the chance of not rolling a 2 when rolling a die" are probabilistic questions. We know the chance of observing heads in a coin flip is 0.5 and we know the chance observing each value when rolling a die. So, when it comes to probability, we have a known model about the world but we haven't got any data yet (we haven't observed anything). I know a fair coin toss can either result in heads or tails [P (heads) = .5] but I don't know which one is going to occur when I toss one.
On the other hand, statistics starts with the data but we do not know about the truth. Whole purpose is to infer the truth by leveraging the data. For example, tweaking the question above,"Observed 8 heads in 10 coin toss, was that coin fair?" is an example of statistical question. I have the data, now I want to know if it is reasonable to act as if P (heads) = 0.5.
There is a distinction within probability: frequentist view and Bayesian view. In an intro class, and more prominently, frequentist view is considered. This approach takes probability as a long-run frequency and when we say probability of observing heads is 0.5, we are referring to the proportion of times we would observe heads if we tossed the coin infinite number of times. Here's an example for intuition:
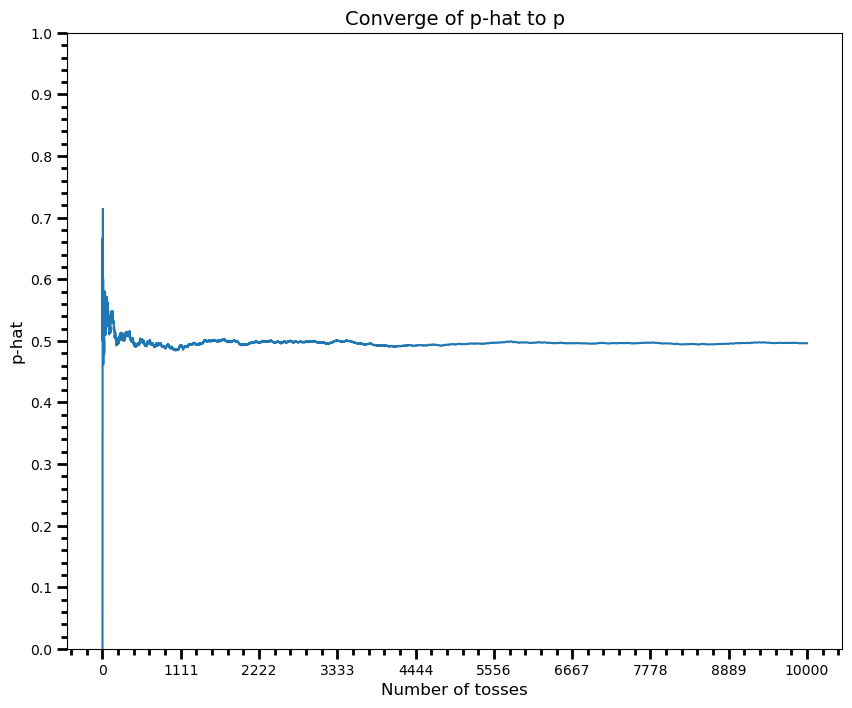
Here one can see the proportion of heads after n tosses. As you see, this proportion converges to the 0.5, probability of heads, as n increases (when probability, as it goes infinity). This is called law of large numbers.
Frequentist view takes probability as a long run frequency, as n goes infinity. However, there is no such thing as tossing a coin infinite number of times: We can't observe infinite sequence of events. Furthermore, a frequentist cannot assign probabilities to non-repeatable events: It wouldn't make sense, and possible, to calculate proportion of interested outcome out of all outcomes. This has some ties with hypothesis testing: we are not able to assign probabilities to hypotheses. For that, one needs to be Bayesian.
Bayesian view takes probability as a degree of belief. In that way, it is subjective: Two people can assign different values. Also, it allows you to assign probabilities to non-repeatable events. Despite these differences, both parts shake hands on the rules of probability.
One thing that bothers me a bit about the two view above is that people, researchers, sound scientists, identify themselves as either Bayesian or frequentist. One does not have to choose side. These are just tools that allow different things (we will discuss this in future posts), use the appropriate one. With that being said, most of the introductory classes (to my knowledge, every one of them) only mentions frequentist tools. So, if you have taken a introduction to stats class before you were probably learning frequentist stuff.
Let's sum up before everything gets out of hand. Probabilistic questions start with a known model of the world, without any data. On the other hand, statistical questions start with the data. From the data, we try to come up with the model or decide whether or not it makes sense to act as if certain model is right.
I hope you enjoyed this piece. This one was largely grounded on Daniel Navarro's Learning Statistics with R. In my opinion, it can be a great bridge from intro to advanced or one can start statistics journey with it if willing to put in some extra hours. Strongly recommended!
Thank you for reading. If you have come this far, you may consider subscribing!
Comments